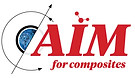

Research Thrust I
Thrust I in Big Picture
To reveal the material-process-microstructure-performance (MP2) relationship over thermoplastic composites’ life cycle, it is necessary to develop a computational framework based on a multiscale approach that can make an efficient and precise prediction of thermo-mechanical behavior of thermoplastic composites by combining the strengths of each of the models in different scales. We refer to this computational framework as digital life cycle (DLC).
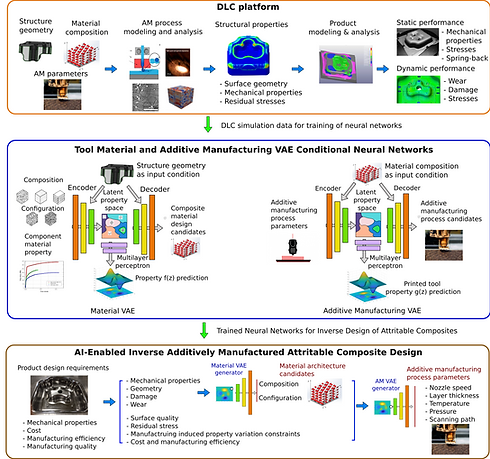
Objectives of Thrust I
-
Establish experimentally validated computational models for high-fidelity analysis of the multiscale, multiphase, multiphysics manufacturing process to predict after-process microstructure and properties.
-
Generate a sufficient amount of data linking the microstructure with the macroscopic thermo-mechanical performance for training and validation of the physics-based AI models.
-
Achieve uncertainty characterization over the entire DLC system as well as assess the decision uncertainty in the manufacturing process.
-
Develop a physics-based modeling approach to enable material and manufacturing design for energy-environment-economy (E3) performance.
Digital Life Cycle (DLC) of Thermoplastic Composites

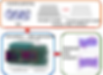
Coarse Grained Modeling of Polymer Matrix-Filler Interaction
-
Stress-strain relationship and strain-hardening & fracture behaviors
-
Effect of molecular structure on strain-hardening modulus and chain extensibility
-
Effect of molecular structure and polydispersity on maximum stress

Microstructure Modeling
-
Develop microscale/mesoscale models that serve as a bridge between multi-physics-based nanoscale models and macroscale material models.
-
Construct 3D RVEs that accurately reflect the microstructure.
-
Developing microstructure models to account for the effect of microstructure/geometry of reinforcements in composite materials.

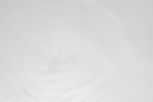
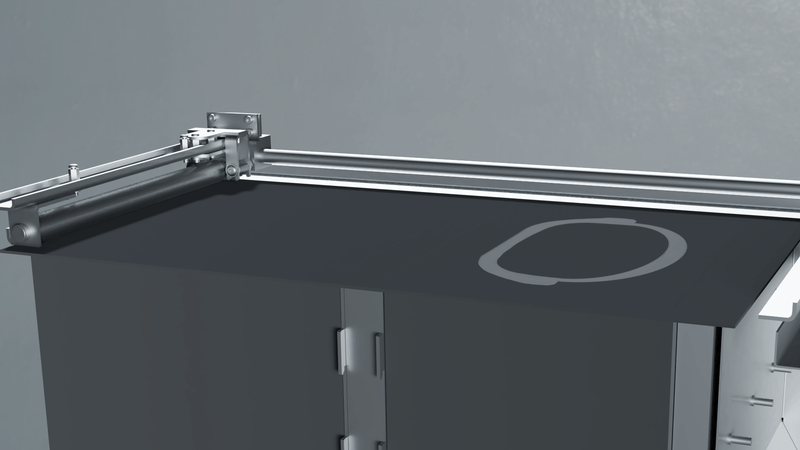
Process Modeling
-
FDM AM Process Modeling - predict void distribution, residual stress in the AM process as a function of AM process parameters and thermo-mechanical properties of the printing polymers.
-
Thermoforming Process Modeling - FE model is developed for performing thermoforming analysis of a composite hat structure to obtain residual stress and spring back resulting from the process.
-
SLS AM Process Modeling - A multiscale selective laser sintering (SLS) additive manufacturing (AM) digital life cycle (DLC) model chain has been developed to capture the behavior of PA12 at different stages of the manufacturing process.
-
M. Athale, T. Park, R. Hahnlen, F. Pourboghrat. “Design, performance, and cost savings of using GF-PC additively manufactured tooling for stamping of HSS 590 sheet metal.” Journal of Manufacturing Processes 101, 1-14, 2023.
-
S. Pradeep, A.Deshpande, P. Y. Lavertu, Z. Ting, V.A. Aditya, A. Yiro, G. Li, S. Pilla, Novel Digital Lifecycle (DLC) for Material-Process-Microstructure-Performance (MP2) relationships of Thermoplastic Olefins Foams Manufactured via Super Critical Fluid (ScF)
Assisted Foam Injection, Polym Eng Sci. 2024 (Minor Revision)

Uncertainty Quantification
In this EFRC task, we aim to develop data- and knowledge- driven statistical models to surrogate the internal structure of the complex system, ensure computationally efficient uncertainty propagation with mathematical guarantees, and facilitate critical decision-making with respect to different choices such as materials and manufacturing setup over the entire
manufacturing process.
-
C. Kerfonta, S. Kim, Y. Chen, Q. Zhang and M. Jiang. “Sequential Selection for Minimizing the Variance with Application to Crystallization Experiments”, The American Statistician, accepted, 2024.
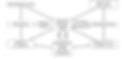


Energy-Environmental-Economic (E3) Analsysis
The goal of this task is to minimize the life cycle impact of material compositions and manufacturing processes conceived in DLC. In this regard, we would like to combine the process modeling theory with the material design constraints in a single model which will help to efficiently design both materials architectures and process steps with minimal carbon footprint. The E3 modeling will undertake a multi-objective optimization (MOO) process combining the DLC material and process models with carbon footprint, process efficiency and economic evaluations.