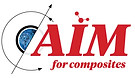

Research Overview
Movivation
-
On the materials side, the most intrinsic and distinctive quality of composites is their degree of heterogeneity and anisotropy, which gives to them a complex range of both physical and chemical properties. The large number of material composition and architectural possibilities result in a vast material design space that has not been fully explored, particularly because the traditional direct method for solving material design problems is largely a trial-and-error process that can be extremely inefficient. Analytical optimization process uses the mathematical model to explore the functional space. When applied to composites, analytical models can only be obtained for the simplest composite architectures such as orthotropic lamina. For more complex material architectures, adding the influence of manufacturing processes, constructing an all-in-one analytical model describing the functional behavior of the composite structure is difficult.
-
On the manufacturing process design side, traditionally, optimizing composite manufacturing processes involved fixing manufacturing process parameters and then experimentally validating the parameters via a substantial number of test coupons to develop a set of “B-basis Allowables” and predict failure. An equivalency test matrix would be completed for changes in the material constituents. Such processes rely on existing domain knowledge. They are expensive, time-consuming, inhibit agility, and represent a substantial barrier to exploring the design spaces and transition of new composite materials and processes. Furthermore, an important characteristic property of composite material structures is that both the material and the structure are created during the manufacturing process. Strictly speaking, composites are not materials; they are more like small-scale structures generated by the material and manufacturing process together. The quality and properties of the final manufactured part depend on the method used to produce it, sometimes as much as the constitutive materials. Hence, manufacturing is a crucial step in the development of products made of polymer composites. In the current practice, material design and manufacturing process development are mostly disconnected, preventing researchers from seizing the opportunities brought by their combined effects.
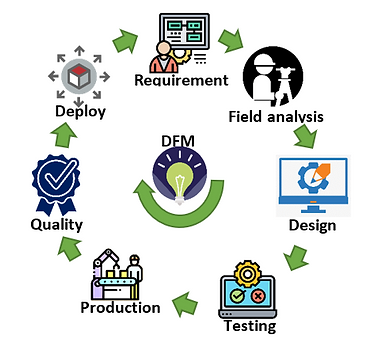
Traditional product development process
Research Goals
-
Unravel the fundamental underpinnings of the material-process-microstructure-performance (MP2) relationship via constructing an “Digital Life Cycle” (DLC) that represents a suite of seamlessly linked, experimentally converged, high-fidelity models embracing all stages of a composite component’s life cycle, linking perceived risk from energy consumption to carbon footprint;
-
Leverage physics-informed AI models and build microservice-based cloud tools to enable inverse composites material architecture and manufacturing process design;
-
Inform and validate the DLC and AI models and implement new material and process designs by exploiting innovative material engineering, characterization, and testing methods.
Knowledge Gaps to Reach Scientific Goals
-
The models revealing the MP2 relationship need to be able to capture the material behavior at multiple length scales (impurities, complex compositions), the effects of manufacturing process (phase changes, non-equilibrium characteristics, E3 impact), and both aleatory and epistemic uncertainties.
-
The possible material architectures and process conditions lead to a vast material and process design space with unknown boundary and few data points, so it is challenging to determine the data sampling strategy and the volume of data to be generated for training ML models.
-
Integration of experimental characterization and testing with model development and validation.
-
How the physical principles will be preserved in ML models for them to represent nonlinear and transient functional properties.
-
E3 impact together with material property, manufacturing quality, and structural performance makes the inverse design multiscale, multi-objective and multidisciplinary.
-
Data fusion and flow among the DLC and ML models, experiments, and the inverse design steps
Research Approach

The scientific goals will be achieved via three research thrusts as shown in the three circles in the figure above. Research Thrust I (green circle) is the DLC representing a suite of seamlessly linked, high-fidelity multiscale models for simulating all stages of a polymer composite’s life cycle, which also integrates uncertainty and E3 impact quantification. The DLC will enable the generation of a large quantity of high-fidelity data for the training of AI models. Equipped with the DLC generated data, in Research Thrust II AI modeling and inverse design research thrust (blue circle), we are developing new AI models, including physical informed neural networks (PINN) and multiscale deep neural operators (DeepONet), to efficiently map the composite materials’ architecture and the manufacturing process to composite components’ performance. Next, we are developing generative neural networks (MaterialGEN) for material inverse design and a conditional VAEGAN neural network (ProcessGAN) for manufacturing process design. By utilizing the experimental facility and capabilities at the Center for Composite Materials at University of Delaware, the Center for Manufacturing Innovation at the University of Florida, the Pacific Northwest National Laboratory (PNNL) and other relevant BES facilities and infrastructure, in Research Thrust III we are conducting material characterization and testing of mechanical, physical, rheological, and morphological properties at nano-, micro-, and macroscales to inform and validate both DLC and AI models and simulations (orange circle). We will also implement new/hybrid processes that combine existing or new scalable processing routes to create tailored composite micro and macro structures. Finally, the inverse design is performed by the generative AI models. For given performance requirements, the material inverse design is first carried out using MaterialGEN to achieve the target material properties. For each material design candidate, and with the quality and E3 impact requirements, the manufacturing process inverse design is performed using ProcessGAN. Thus, the optimal composite material and its manufacturing process is obtained as a holistic solution.
Synergy
The EFRC program is not a simple conglomeration of all the expertise and resources. While the research team is grouped into DLC, AI and Experiments research thrusts according to their functions, the scientific goals will be achieved through the collaboration of the research thrusts. Each research thrust not only provides information to but also validates results for the other two. The three research thrusts form an iterative loop and the iteration among them continues until all three produce consistent results and thus the research has converged. Therefore, combining all the expertise and capabilities of the team together, and with this helical collaborative iteration, we believe the scientific challenges can be addressed and the overarching goal of the project can be reached.

Collaborative effort to address the challenges in the proposed interdisciplinary research. Orange column: research challenges of the project; blue column: disciplinary expertise needed to address the challenges; green column: facilities/resources required for the research activities; purple column on the left: EFRC’s domain experts in the required disciplines; purple column on the right: EFRC team’s resources to support the research activities.